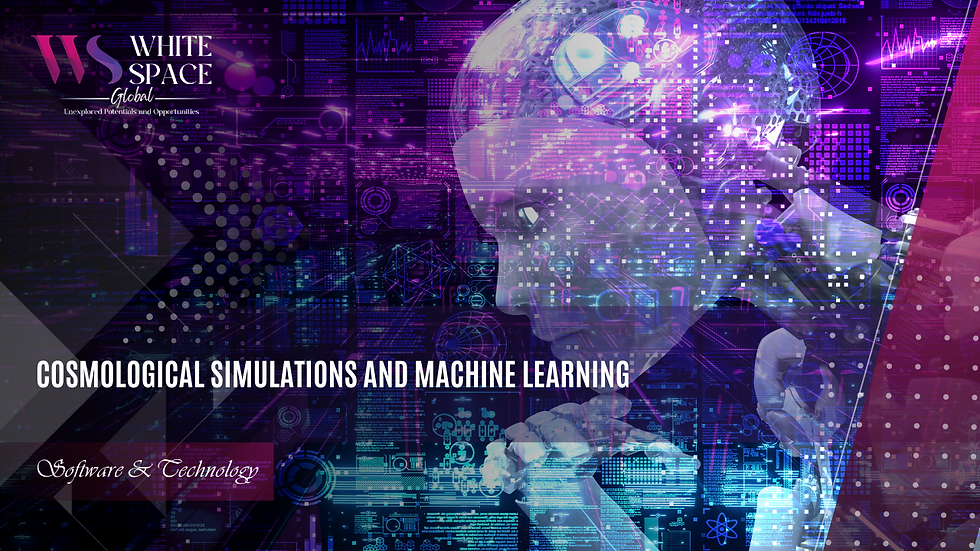
What are Cosmological Simulations?
Cosmological simulations are essential tools in modern astrophysics to understand the complex structure and evolution of the universe. These simulations involve solving a large set of equations to model the behavior of the universe's fundamental particles and their interactions. Cosmological simulations use computational techniques to study the evolution of the universe.
These simulations involve modeling the behavior of fundamental particles, such as dark matter and gas, as they interact over billions of years. The resulting simulations can be used to predict the distribution of matter in the universe, the formation of galaxies, and other phenomena observed in the cosmos. However, the massive amount of data generated by these simulations makes it challenging to analyze and interpret the results.
Machine Learning and Cosmological Simulations:
Machine learning algorithms offer a solution to this problem by enabling researchers to extract valuable information from large cosmological simulations. These algorithms are trained on a subset of the data generated by the simulation and learn to identify patterns and relationships within the data. Once trained, they can be used to analyze and interpret the vast amounts of data generated by the simulations, providing insights into the structure and evolution of the universe.
There are several areas in which machine learning is being applied to cosmological simulations:
Dark Matter Halos
Dark matter is a mysterious substance that makes up approximately 85% of the universe's matter content. Cosmological simulations can predict the distribution of dark matter in the universe, but identifying individual dark matter halos is challenging. Machine learning algorithms can be used to identify and classify these halos, providing insights into the structure of the universe on large scales.
Galaxy Formation
Galaxy formation is a complex process that involves the interplay between dark matter, gas, and other forms of matter. Cosmological simulations can model this process, but the vast amount of data generated makes it challenging to identify the physical processes that drive galaxy formation. Machine learning algorithms can be used to analyze these simulations and identify the physical processes that are most important in determining the formation and evolution of galaxies.
Gravitational Lensing
Gravitational lensing occurs when the gravitational field of a massive object, such as a galaxy cluster, bends and distorts the light of objects behind it. This distortion can be used to study the distribution of dark matter in the universe. Machine learning algorithms can be used to analyse large datasets of gravitational lensing observations, providing insights into the structure of the universe on large scales.
Conclusion
Cosmological simulations and machine learning are two essential tools in modern astrophysics. By combining these techniques, researchers can extract valuable insights from large datasets of cosmological simulations, providing a deeper understanding of the structure and evolution of the universe. As computational power continues to increase, and machine learning algorithms become more sophisticated, we can expect to see further advances in our understanding of the cosmos.
Comments